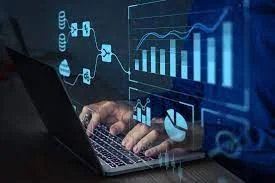
Gartner’s recent report on the state of the data analytics industry suggests a number of exciting trends to watch. By 2022, Gartner expects 35% of large organizations to sell or buy data in formal online data marketplaces. The trend of cloud-based data services is expected to accelerate. But how will all of these new technologies impact the industry? Here are some predictions:
Data as a Service
Graph processing and graph database applications are predicted to grow at a staggering rate over the next few years. These technologies will enable more complex and adaptive data science. Gartner analyst Amir Sallam noted that augmented data management and data fabric are closely tied. They allow different tools to access data more efficiently and integrate data management processes. By 2026, these technologies will widely adopted. Here are four trends in the data analytics industry:
Consumer data will the most important differentiator in the next two to three years. Many companies do not have the budget or negotiation power of large organizations. Consequently, these companies need a cost-efficient way to process and analyze data. Data democratization and data integration have also expected to increase in the coming years. Ultimately, this will increase efficiency and reduce potential human error. For now, the industry is primarily focused on enterprise-level analytics, but the trend toward democratized data analytics will continue to rise.
AI
With the introduction of COVID-19, the world has experienced a global disruption that has disrupted every enterprise. As a result, many enterprises have trying to prepare themselves for the disruption that is likely to come in 2022. Fortunately, AI can help with this disruption. In addition, the development of no-code AI systems can enable companies to make use of powerful AI systems without the assistance of data scientists. This will reduce costs and enable non-experts to build applications with ease.
In recent years, the adoption of graph technology has increased, which is the foundation of modern data and analytics. Graph technologies enable easier user collaboration, more accurate machine learning models, and more explainable AI. These technologies have been available for some time, but have now becoming increasingly commonplace in the data analytics industry, with Gartner estimating that 50% of client inquiries will involve some sort of graph technology. Previously, business users have limited to manual data exploration and predefined dashboards. These dashboards have often created by citizen data scientists.
Machine learning in data analytics industry
There are several major machine learning trends in the data analytics industry. In the field of customer support, machine learning has used to develop chatbots and recommendation engines that mimic human customer service actions. These systems provide real-time assistance and offer personalized user experiences, learning from their interactions with customers and delivering strategic demographic data to data scientists. In addition, machine learning can help companies improve fraud detection and streamline their supply chain. But the most important trend in this industry is that it’s only beginning.
Machine learning algorithms have used to improve user experiences by analyzing speech and text. Companies can now use text and voice analytics to provide accessible captions. The field of machine learning in the data analytics industry is exploding. Machine learning is crucial for self-driving vehicles and has many uses beyond these. It is also a vital part of sensor applications such as analyzing geographic data to predict which location will yield the best renewable energy.
Graph analytics
Predictive and prescriptive analytics have already on the rise in the data analytics industry. But what has the next big step? Graph processing and graph databases are growing at 100% per year, according to Gartner’s forecast. These technologies have also helping companies improve customer journeys, by providing a user-friendly interface for data analysis. They’re also helping companies upskill employees, and enabling them to leverage data in new and better ways.
Context-enriched analytics have based on graph technologies and can help organizations identify users’ needs, constraints, paths, communities, and more. Graph analytics have also helping organizations identify fraudulent activities. For example, a fitness application could merge health news feeds with data about a person’s physical activity. Then, they could predict how diseases spread. And when a Trend like this hits the market, it will make it possible to take action.
Edge computing
There are two main types of edge computing systems. The first type of edge system is purchased on the business end and deployed within the company. This would involve selecting the right edge devices, implementing the network architecture, and purchasing management software and expertise to deploy the system. The latter has typically reserved for large organizations. In both cases, edge computing is a promising technology for businesses seeking to improve operational efficiency and reduce costs. It has a rapidly growing industry that promises great potential in the data analytics industry.
The second type of edge computing is called a decentralized system. The advantage of deploying edge computing is its ability to eliminate latency and increase speed. For example, if a train is running, a train sensor can provide data about its engine status without having to send the data to a central data center. This means data from a train sensor can processed more quickly. It can also reduce costs and improve security.
Supply chain analytics
Supply chain analytics have a form of predictive analytics that help businesses make better decisions by using historical data to identify patterns and predict future events. These methods use embedded decision logic to identify patterns and make smarter decisions, such as the location of a factory or how to handle retail shipment strategies. By using predictive analytics, businesses can avoid costly mistakes and improve their supply chains. In this article, we will explore the latest trends in supply chain analytics and how they can benefit your business.
The main barriers to implementing supply chain analytics include finding the right people and financial resources. Without the proper people and resources, analytics programs will fail to yield any results. As a result, leaders and executives must support and encourage analytics. Here have some of the challenges and benefits of supply chain analytics: